|
History of NSF funded grants
History of NASA funded grants (Enter "Foufoula" under Principal Investigator)
Active Research Grants
Current research funding for the group is from the US National Science Foundation (NSF) Division of Information
and Intelligent Systems under the ExpandAI program (Grant IIS2324008) and by NASA through the Global
Precipitation measuring Mission (GPM) Program (Grant 80NSSC22K0597), the Weather and Atmospheric Dynamics
Program (Grant 80NSSC23K1304), the Commercial SmallSat Data Scientific Analysis (CSDA) Program (Grant
80NSSC24K0046), and a student fellowship under the Future Investigators in NASA Earth and Space Science
Technology (FINESST) Program (80NSSC24K0033). Research is also supported by the UK Research and Innovation
Global Challenges Research Fund (GCRF) under the Living Deltas Hub (Grant NES0089261).
Please click an award to learn about our research:
ExpAI2ES: Expand AI2ES for 4D space-time organization of precipitation processes and extremes,
visualization tools, and workforce development (NSF Grant IIS2324008)
ExpAI2ES is a partnership of San Diego State University (SDSU), University of California Irvine (UCI) and
the NSF AI institute AI2ES ("AI Institute for Research on Trustworthy AI in Weather, Climate and Coastal
Oceanography") at the University of Oklahoma (UO). The research focus of ExpAI2ES is on AI/ML
modeling of the space-time organization and multiscale structure of precipitation and other
atmospheric variables, with special emphasis on extremes and uncertainty quantification. Both SDSU
and UCI have a solid foundation in AI research and education, as well as a joint graduate program in
Computational Science. ExpAI2ES will scale-up these efforts and advance research on explainable AI for
precipitation extremes and their uncertainty, develop engaging visualization tools for research and
student and teacher training, and bring to the workforce a diverse and untapped talent pool through
sustainable collaborations with AI2ES.
As part of this project, we will explore deep neural networks (DNN) for probabilistic learning of
multiscale features in precipitation fields and extreme storms. The input data will include dynamical
multispectral observations of cloud systems as observed from geostationary satellites at high spatial and
temporal resolutions (1~5 km, 5~30 min), satellite quantitative precipitation estimation products, and
reanalysis data, which are all globally available, as well as regional forecast outputs. The network
architecture will be chosen for its capability in extracting information from the inputs across a wide
range of scales, and, for the cloud data in particular, to extract dynamical information characterizing the
evolution of cloud systems over space and time. A Mixed-Scale Dense Deep Convolutional Neural
Network (MSDNet) will be an architecture to start with (see preliminary results below and Guilloteau et
al. 2023), although other architectures will be explored.
At the same time, uncertainty quantification (UQ) of extremes in global observational or modeling
datasets is needed for risk estimation and for the data service to users and decision-makers. Yet, UQ is
still lacking in these products, and even if available, it is restricted at "the grid scale" which might differ
from the size of a hydrologic basin where decisions need to be made. Obviously, UQ estimates do not
scale-up trivially and novel methodologies are needed to provide bounds of extremes at a range of
scales of interest. As part of the ExpAI2ES collaborative research, we will explore the use of quantileregression probabilistic ML techniques to predict the probability of occurrence of precipitation extremes
at various scales, from existing models and weather observational records (knowing their systematic
biases, inaccuracies, and uncertainties in the representation of precipitation extremes).
The probabilistic ML framework will be used as a basis for stochastic ensemble generation,
constrained so that they respect the true statistical properties and spatiotemporal organization of
precipitation across scales. In our previous work we explored the preservation of the multiscale
properties and structures of precipitation fields by imposing specific constraints in the cost function of a
deep neural network. It was shown that constraining the statistical distribution of precipitation rates at
the pixel scale is insufficient to preserve the statistical properties and structure of the precipitation field
across all scales; however, a multiscale constraint can efficiently be imposed on the wavelet power
spectrum of the field, yielding improved performance (see Figure). This work will be extended and
embedded into the probabilistic framework of ensemble generation.
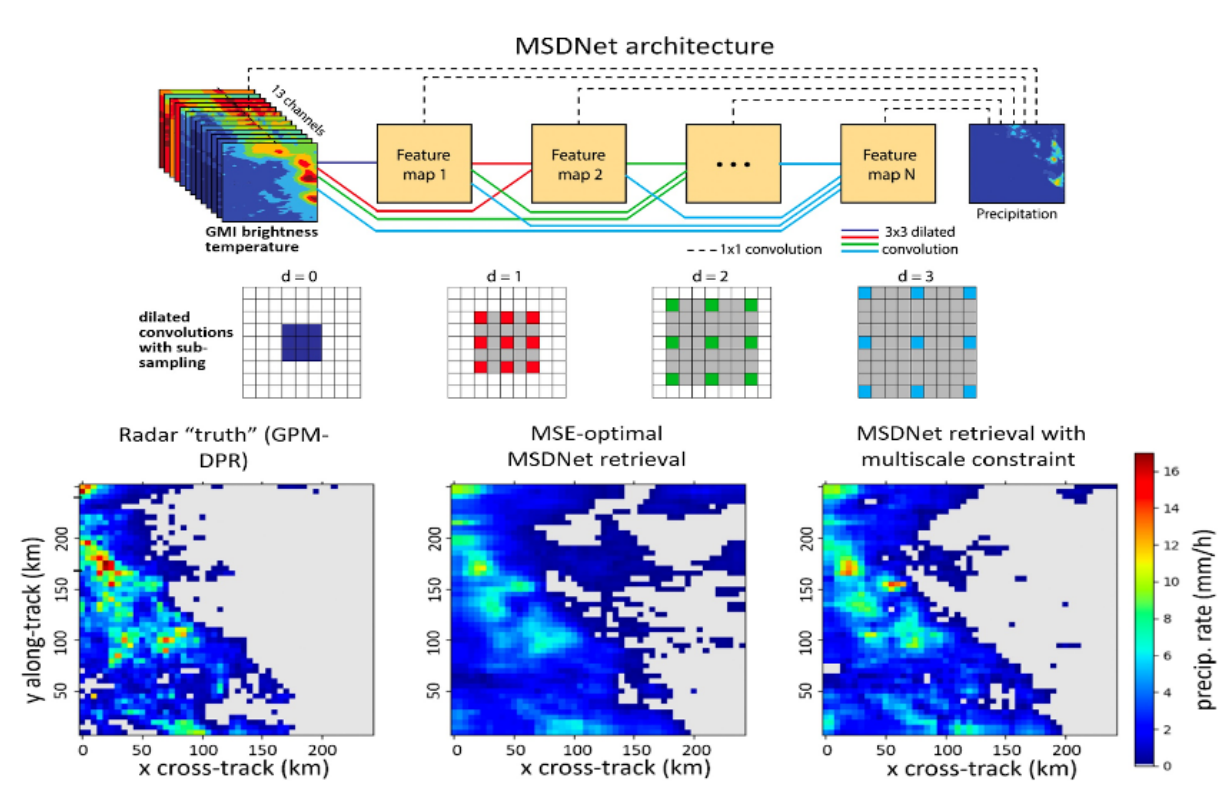
(Top) Simplified representation of a MSDNet architecture. The dilated convolutions with subsampling
allow keeping the size of the convolution kernels constant (3x3 pixels here), enabling dense connections
between all the MSDNet layers. (Bottom) Two examples of MSDNet retrievals trained with different loss
functions. For details see Guilloteau et al., 2023.
River response to climate change: insights from high-resolution remote sensing data in High Mountain
Asia (NASA Grant 80NSSC24K0046)
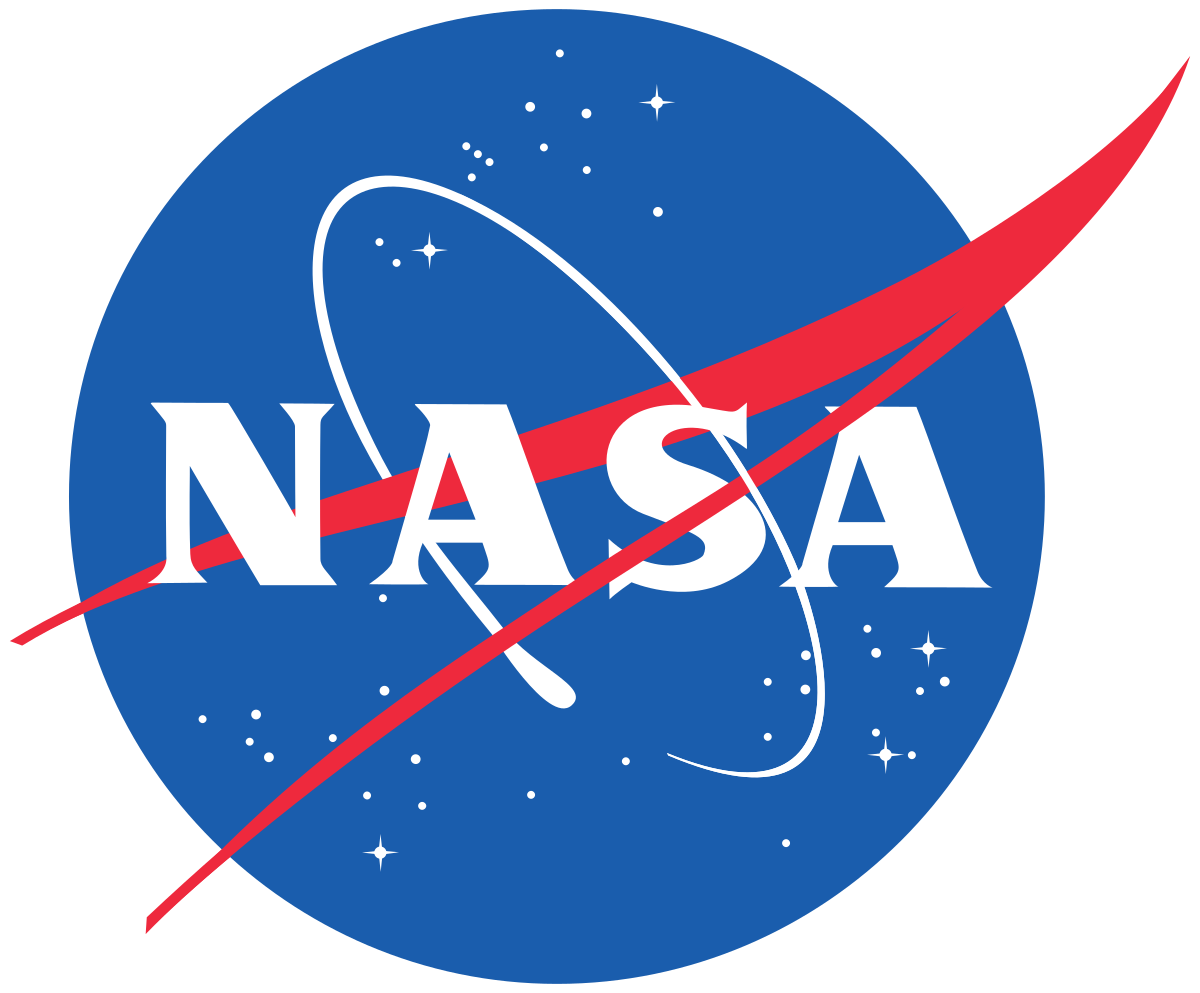
Climate change poses an urgent global challenge for river systems. Rivers are experiencing drastic
alterations to streamflow, sediment supply, and base level due to rising temperatures, increased
precipitation, melting glaciers, and other climate drivers. These changes may alter the geometry of
rivers, affecting their evolutionary trajectory and endangering nearly three billion people worldwide
who live along river corridors. Theoretical and experimental models indicate that an increase in water
and sediment discharge should leave a distinct imprint on rivers. For example, increased surface runoff
is predicted to widen river channels and create more numerous sub-channels. Climate change can also
affect river mobility: increased sediment supply and sea-level rise are predicted to accelerate riverbank
migration and increase the frequency at which rivers jump course across the floodplain. Testing these
predictions is difficult with conventional field studies because rivers can take years to respond to
external perturbations. Consequently, we lack observational evidence for how climate change affects
channel geometry and channel mobility. Addressing this knowledge gap is crucial for sustainably
managing water resources in the coming decades, and for protecting people and property on river-
adjacent land from flooding and erosional hazards.
In this project we will leverage NASA's Commercial Smallsat Data Acquisition (CSDA) program to
evaluate climate-change impacts on river channel geometry, configuration and mobility, with a
geographic focus on the High Mountain Asia. HMA provides an exceptional natural laboratory for the
proposed work based on three criteria. First, rivers in HMA have been subject to persistent increase in
sediment and water supply since the mid-1990s because of melting glaciers and a shift towards warmer
and wetter climate. Second, HMA is geographically remote; these rivers are relatively undisturbed by
human activity (e.g., dams, agriculture, levees), allowing us to isolate river response to climate change.
Third, HMA rivers are expected to rapidly respond to climate change because the aridity and steep
terrain of HMA promote high sediment fluxes, narrow channel widths, and limited vegetation-factors
that facilitate a relatively short channel-response time (months to multiannual timescales) compared to
lowland coastal rivers (decadal to millennial timescales). Thus, HMA rivers offer a first glimpse of
changes yet-to-come for other, more densely populated lowland river systems. HMA will serve as a
valuable testing ground for predictive models, setting the foundation for successful predictions of
climate-change impacts on rivers worldwide.
We will use the wealth of observational data available from the CSDA RapidEye, IKONOS, and
EarthDEM assets and state-of-the-art techniques for quantifying river configuration (i.e., entropic
Braiding Index developed in our group; Tejedor et al, 2022) and channel mobility (i.e., particle image
velocimetry) through time to document changes in channel geometry and channel mobility in HMA from
the late 1990s to present (see Figure). We will focus our analysis on 29 river basins for which climate-
induced changes in sediment supply, water discharge, and downstream lake level are documented (Li et
al., 2021, 2022) . We plan to test 2 hypotheses: (H1) Climate change is causing channels to adjust to
wider geometries and more complex configurations and (H2) Climate change is accelerating the pace of
river channel mobility.
Enhancing the Representation of Precipitation Space-Time Dynamics in Satellite Products:
Validation, Error Modeling and Improvement of Retrieval Algorithms (NASA Grant
80NSSC22K0597)
The space-time dynamics of precipitation, and in particular the sub-mesoscale dynamics, play an
important role in hydrologic modeling and prediction. Numerous studies have underlined the fact that,
because runoff is a strongly nonlinear process, for basins of any size, the hydrologic response to
precipitation depends not only on the precipitation average over the basin but is also strongly dependent
on the spatio-temporal structure of the precipitation systems. Some "extreme" and catastrophic events are
characterized by a peculiar dynamic rather than unusual amounts of precipitation (e.g., stationary storms
causing catastrophic floods).
The goal of this project is to answer the following questions: (1) What exactly space-time structured
variability are satellite precipitation products not able to reproduce, how does this differ over different
precipitation regimes and how does this depend on ancillary environmental variables that can be extracted
from synergistic geostationary and low-orbit satellite observations? (2) How can this knowledge be mined
to develop quantitative scale-dependent error models which can be used to inform end users, guide multi-
sensor merging and ultimately improve the accuracy of global satellite precipitation products? and (3)
How can the multi-scale space-time structure of observed brightness temperatures, and of the underlying
precipitation process, be explicitly incorporated into the next generation of Machine Learning (ML)
precipitation retrieval algorithms?
The specific objectives of this project are:
Objective 1: Develop and implement a framework for space-time satellite precipitation error
diagnostics, uncertainty quantification and error modeling, across space-time scales relevant to climate,
weather and hydrological applications. The innovations lie in (a) undertaking a comprehensive
assessment of satellite products' capabilities and limitations in reproducing the precipitation space-time
dynamics at all scales relevant to climate analysis of precipitation dynamics and hydrologic applications
at the basin scale (see Fig 1); and (b) capitalizing on this assessment to develop a scale-dependent
stochastic error model that systematically associates a quantitative measure of uncertainty to each
satellite estimate, to inform end users and for optimal merging of multi-source information (see Figure
2).
Objective 2: Improve existing operational retrieval algorithms and develop new algorithms specifically
geared toward enhanced representation of the space-time dynamics of precipitation. The innovations lie
in (a) explicitly incorporating constraints on the multi-scale space-time dynamics of precipitation in the
current operational algorithms, both at the brightness temperature inversion level and at the multi-
source merging level; and (b) developing new Machine Learning (ML) algorithms which are trained to
"look beyond the pixel" by extracting pattern information through multiscale convolution operators, and
by adopting objective functions that aim at preserving the multi-scale space-time structure of
precipitation.
Dynamical Error Modeling and Uncertainty Quantification for Remotely-Sensed Atmospheric
Variables (NASA Grant 80NSSC23K1304)
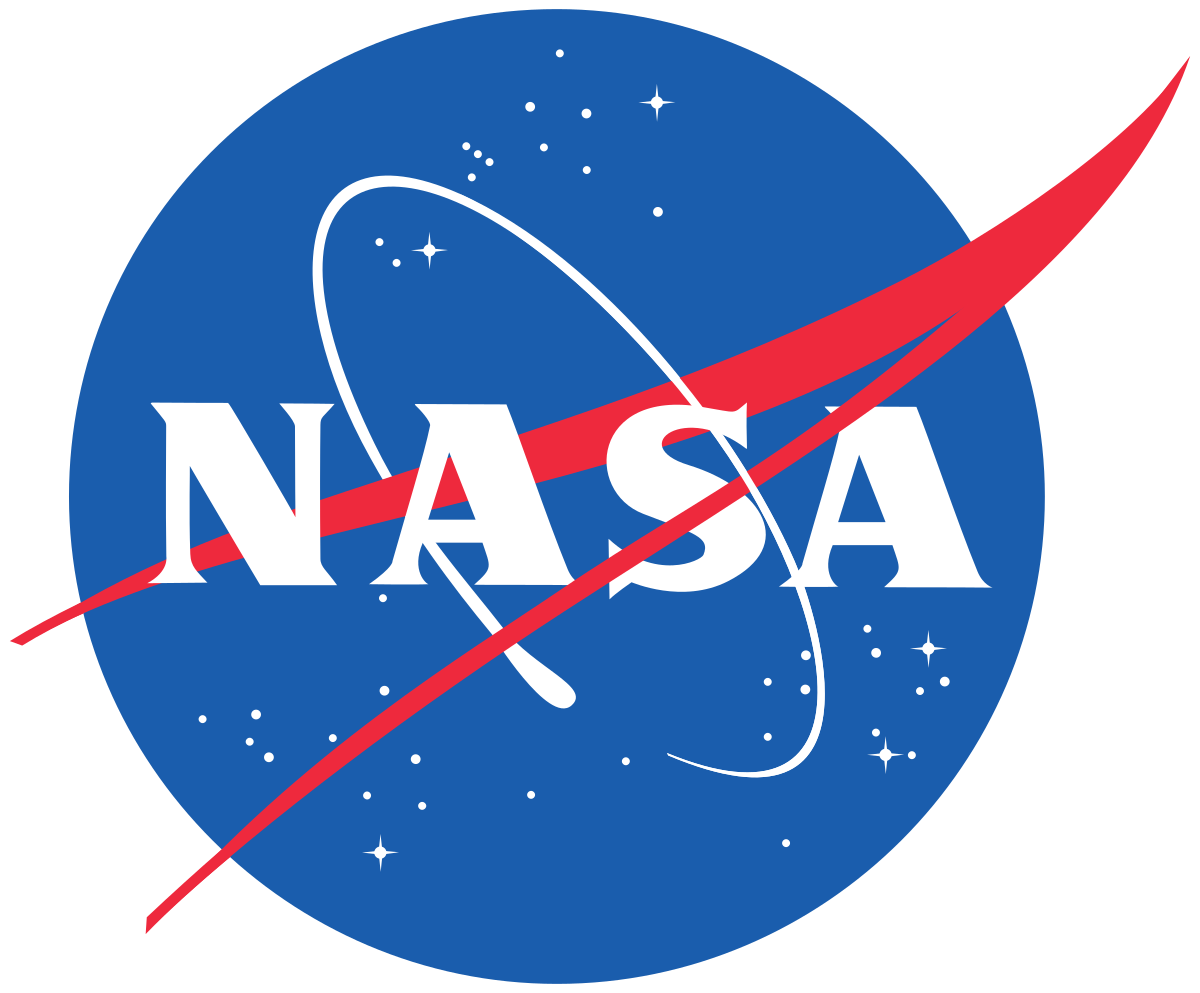
A significant amount of research has been focusing on attempting to provide a quantitative
measure of the uncertainty associated with each individual estimate of remotely-sensed atmospheric
variables. This uncertainty quantification is rendered non-trivial by the heteroscedastic nature of the
estimates, due to the fact that the estimation accuracy is not solely driven by the instruments'
characteristics, but also by the specific observational and environmental conditions associated with each
individual measurement. This heteroscedasticity also inevitably renders the retrieval errors spatially and
temporally correlated. Indeed, if the target variable shows dynamical features and complex multi-scale
space-time organization and patterns, so does the retrieval error. However, when it comes to evaluation
of retrieval performance, uncertainty quantification and error modeling, the majority of studies focuses
on sample statistics, i.e. on the statistical distribution of the retrieval errors at the pre-defined fixed
spatio-temporal scale corresponding to the retrieval's pixel (or voxel) scale. This pixel-wise statistical
characterization ignores the spatial and temporal correlation of the error, its multi-scale structure, and
more generally all the cross-sample (cross-pixel) dependances within the error field.
In this project we propose to go beyond this simple pixel-wise uncertainty analysis and error
modeling approach by developing and implementing advanced uncertainty quantification and error
modeling methodologies that acknowledge the complex state-dependent and scale-dependent nature
of the uncertainty in remote-sensing estimates of atmospheric variables (see Figure). Specifically, the
objectives of this research are:
Objective 1: Provide a global quantitative assessment of the accuracy of remotely-sensed atmospheric
variables (temperature, pressure, humidity, precipitation, and wind velocity and direction) across all
relevant spatio-temporal scales;
Objective 2: Develop a prognostic error model capable of predicting the retrieval uncertainty associated
with each individual estimate, globally, and at any desired spatio-temporal scale. The parametrization of
this error model should be dynamical and locally-adaptative to account for varying environmental and
observational conditions; and
Objective 3: Demonstrate the usability of the developed error model for the stochastic generation of
ensembles from the observations. Each ensemble member will be a possible realization of the true
atmospheric state, given the uncertainty associated with the observations, and it should respect the
true multi-scale space-time statistical properties of the retrieved process.
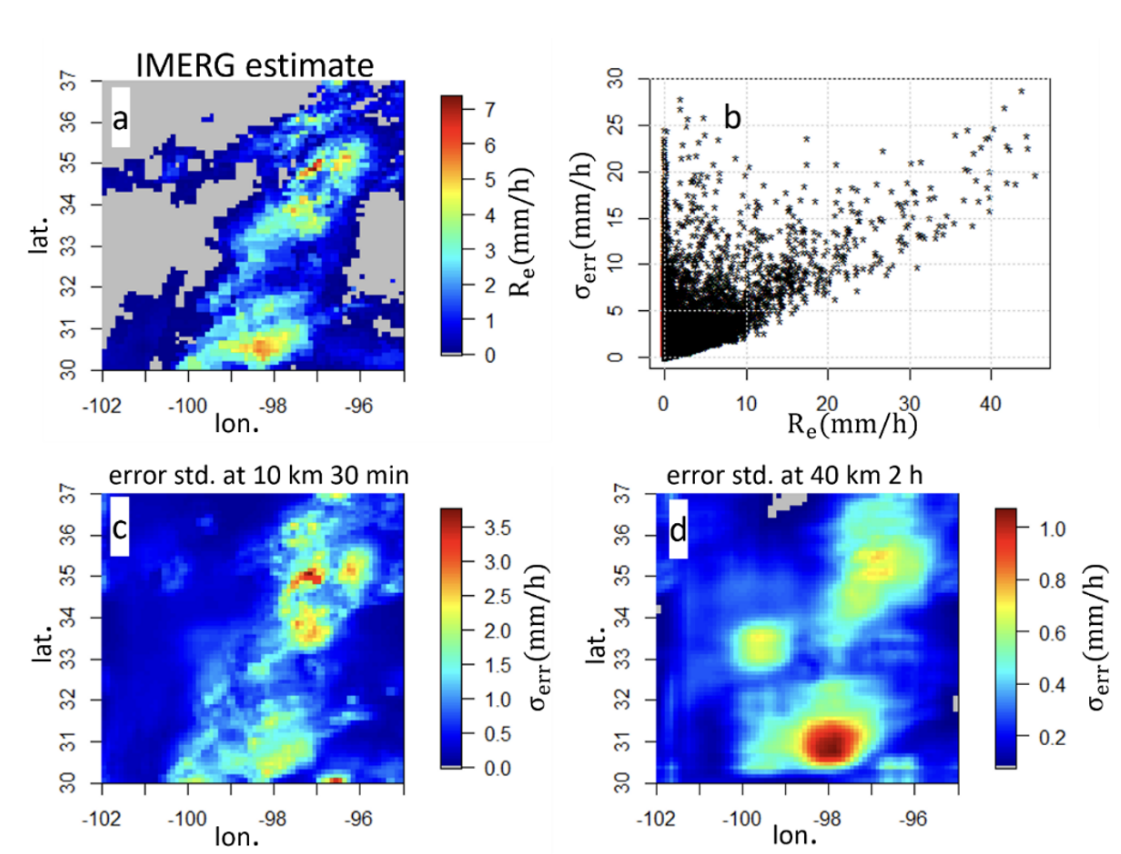
Figure: (a) An example of IMERG retrieved precipitation intensity , (b) Scatter plot of the predicted standard
deviation of the IMERG error from the developed spectral error model vs. IMERG estimated precipitation intensity
at 10 km and 30 min resolution, for the same area as in panel (a). Note that two pixels with the same intensity do
not necessarily have the same standard error, as this error depends on the neighborhood within which a pixel is
embedded, i.e., the multi-scale structure of the field. Map of the predicted standard deviation of the IMERG error
at 10 km and 30 min resolution (c) and 40 km and 2h resolution (d).
Please click an award to learn about our research:
Center for Ecosystem Climate Solutions (CECS)
The Center for Ecosystem Climate Solutions is combining the expertise from eight research institutions to develop and communicate new solutions to managing California's natural lands for climate change.
Our research focuses on how land management practices can lessen the incidence and severity of wildfires in California, while simultaneously enhancing watershed health. We approach this research through an interdisciplinary multi-phases analysis, which includes 4 separate, but interrelated, sub-projects.
These sub-projects are:
1. Mapping Ecosystem Properties and Services
Sub-project 1 will develop the remote sensing and geospatial tools which will consistently quantify and integrate the effects of past and ongoing management on four critical characteristics of the land surface.
2. Determining Management Effects
Sub-project 2 will mine the geospatial data sets and approaches created in Sub-project 1 to determine the effects of past and ongoing management on carbon, wildfire spread, water balance, and vegetation drought resilience.
3. Informing Adaptive Management
The overall goal of Sub-project 3 is to co-develop, test and refine approaches to inform adaptive management of wildlands in ways that enhance carbon storage and build public support for the needed investments and management actions.
4. Valuing and financing carbon storage
Sub-project 4 will support valuation of different services, costs and net benefits across forests, shrublands and rangeland to inform public and private investments in multi-benefit landscape management.
The result of this research and collaboration will be an interactive tool that will assist land managers in their decision-making process. We hope this tool will make these decisions easier, as well as lead to better outcomes for ecosystem health. Please click here to learn more about our research.
NSF: TRIPODS+X:RES - Collaborative Research: Data Science Frontiers in Climate Science
We aim to develop novel machine learning (ML) and network analysis methodologies that facilitate discovery, characterization, and understanding of climate modes of variability and change, and to use this enhanced understanding to improve seasonal-to-subseasonal forecasting.
Our work has two main objectives: (1) Develop novel classification and regression tools that account for highly correlated climate features or covariates, nonlinear interaction terms in high-dimensional settings, and nonstationarity in the climate observations; and (2) Develop network identification methods that leverage recent advances in machine learning and statistics but account for trends and the limited time frame of climate data, and use network representations to analyze the learned dependencies to contextualize and interpret them physically.
To account for the highly correlated covariates in the climate system (for example, Sea Surface Temperatures, SSTs, in the Pacific ocean in space and time), a new regularization approach is developed, called the Graph Total Variation (GTV). GTV is guided by the space-time correlation structure of the covariates to inform a graph-based regularizer, in order to promote similar weights for highly correlated predictors, enforce sparsity and unravel the most explanatory predictive features without specifying them a-priori. To address the issue of the short observational record and robustly estimate the strength of correlations among the predictor variables, we make use of a large ensemble of climate model outputs. This unconventional way of using climate model outputs has recently been highlighted to be highly efficient in reducing the structural uncertainty in the predictor variables, and we aim to further explore the potential of such a utilization. A schematic of the research framework we are developing is shown below, focusing on the case study of regional precipitation prediction in the Southwestern US (SWUS) using climate variables of the Pacific basin.
The TRIPODS+CLIMATE project is an interdisciplinary research effort involving hydrologists, climate scientists and data scientists from three institutions: Efi Foufoula-Georgiou, James Randerson, and Padhraic Smyth (UCI), Rebecca Willett (University of Chicago), and Stephen Wright and Garvesh Raskutti (University of Wisconsin, Madison).
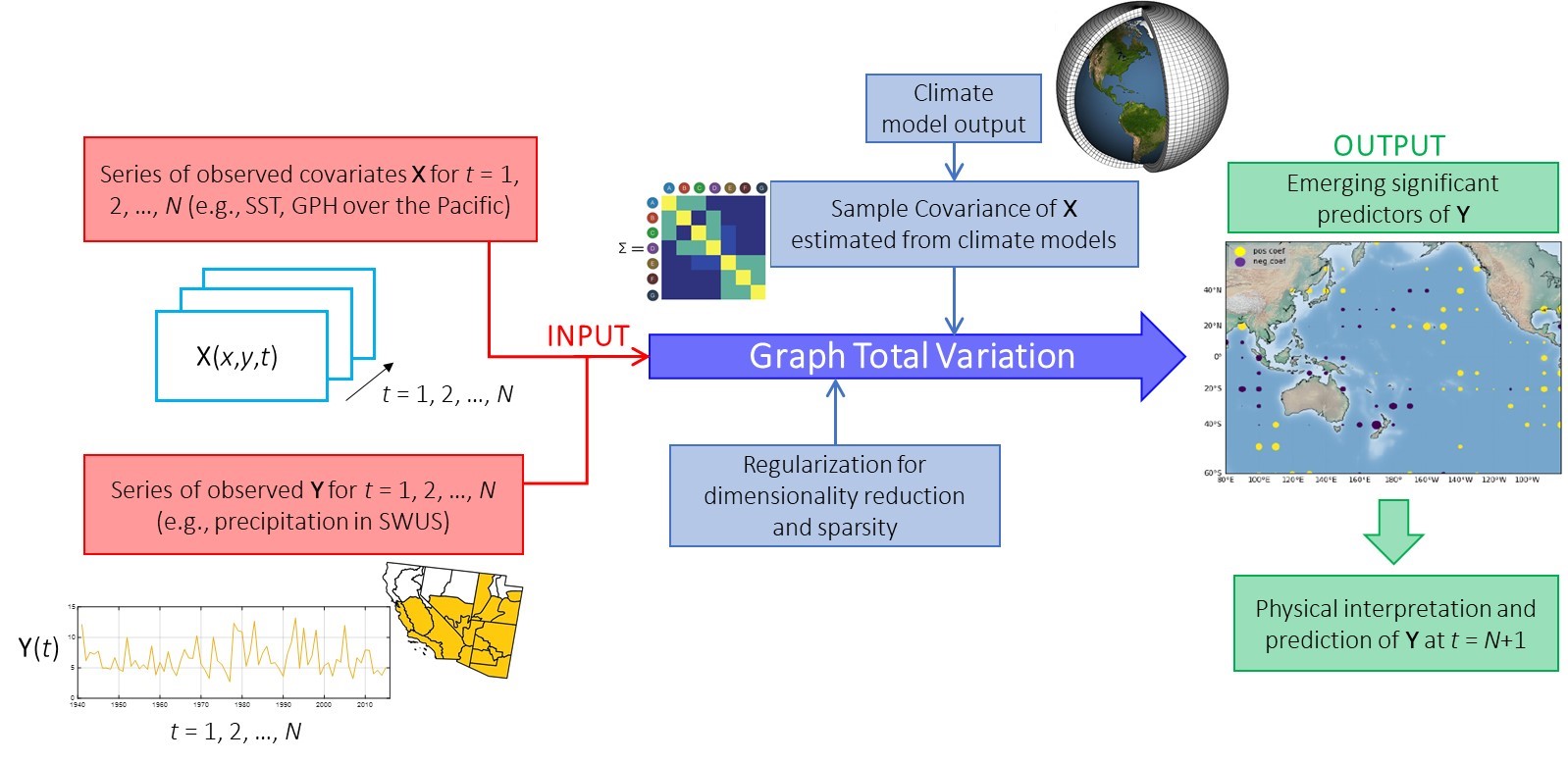
Mining observations and climate model outputs for improved understanding and regional hydro-climate prediction. Graph-guided regularization for predicting winter precipitation over SWUS. We use observed Pacific SSTs to inform our prediction, and we form a space-time covariance graph with edge weights corresponding to pairwise correlations to constrain our regularization scheme. Correlations are estimated based on the output of climate models towards improving the estimation of the dependencies of the predictors and increasing the overall performance of the algorithm. Emerging significant predictors are analyzed for physical interpretation and attribution.
NSF: Collaborative Research - Understanding deltas through the lens of their
channel networks
River deltas are complex eco-hydro-geomorphologic systems exhibiting a large variability in their morphology and in the configurations of the channels that tile their deltaic surface. These channels dictate how water, sediment, and nutrients are spread over the delta top and how they are delivered to the receiving water bodies, and thus are key for delta self-maintenance and resilience to external perturbations. Yet, while tributary channel networks have been exhaustively studied over the past 50 years, the study of Delta Channel Networks (DCNs) is still in its infancy.
As part of this project, we seek to undertake a comprehensive study of deltas through the lens of their delta channel networks. Our hypothesis is that DCNs encode information about the processes that created them and thus quantitative analysis of deltas of different forcings and climate environments through metrics that rigorously capture DCN topology and flux organization, can be used to reveal process from form, classify deltas based on DCNs, explore optimality principles behind delta self-organization and advance our understanding of delta response to change. Analysis of 60 field deltas of diverse climatic, geologic and ecologic environments, and more than 100 numerically simulated deltas under controlled conditions will provide a large database on which to study DCN typologies, self-organization principles, and propose a DCN-based delta classification framework.
Specifically, the objectives we plan to pursue are:
Objective 1: Create a global database of DCNs -- Extract the channel networks of 60 deltas from diverse climatic, geologic, and ecologic environments to generate a global database of delta channel networks for a comprehensive study of their topology and flux distribution;
Objective 2: Explore DCN typologies and relation to process -- Use the database of field deltas and numerically simulated deltas from the Delft3D model to study the topology and flux distribution of their DCNs and propose a set of sufficient metrics that can discriminate between deltas formed by different underlying processes; and
Objective 3: Test DCN optimality and use it for process inference and uncertainty bounds on metrics for enhanced classification -- Use the extensive database of field and numerically simulated deltas to test the hypothesis of an optimality principle behind delta self-organization, understand reasons for deviations from this optimality in specific deltas, and use it to build confidence intervals on DCN metrics that can enhance classification potential.
Deltas have a wide range of morphologies. From top left corner clockwise: Ebro, Parana, Selenga and Wax Lake deltas.
The set of 60 deltas selected for study and for generation of the DCN global database. Most of these deltas have been studied by others in terms of processes, vulnerability to change, etc. but very few, if any, in terms of the structure of their channel networks in an absolute and a comparative sense.
NSF Geomorphology and Land-use Dynamics project EAR-1811909.
NSF: EAGER - Real-Time: Search for dynamical dependencies and natural time-scales of physical processes
NSF EAGER project ECCS-1839441.
NASA: Improving GPM passive microwave retrieval and multi-sensor merging: a nonlocal formulation accounting for the 3D structure of rain
Currently, the state-of-the-art GPM precipitation retrieval algorithms perform the inversion of the microwave brightness temperature (TB) for each pixel (i.e. for each beam position) independently. The spatial consistency of the retrieved precipitation across nearby pixels is neither assumed nor constrained. Moreover, the spatial structure and covariation of the TBs (which reflect the 3D organization of the precipitating system) are not exploited in the inversion. Our hypothesis is that taking advantage of the spatial organization of precipitation and TBs can substantially improve the retrieval from passive microwave sensors and the quantification of the retrieval error for incorporation in multi-satellite merging algorithms.
The goal of this research project is to develop and test a “nonlocal Bayesian retrieval methodology” by extracting relevant information from the spatial variations and co-variations of the TBs at all frequency channels, to overcome the limitations of the pixel-wise approach and increase the accuracy and resolution of the retrieved surface rain. The proposed nonlocal approach is expected in particular to enhance the ability to retrieve the fine scale spatial patterns of precipitation, allowing the retrieval of sharp spatial gradients when the fine scale spatial heterogeneity is strong. In other words, the nonlocal approach is expected to improve the “effective resolution” of the retrieval, i.e., the smallest scale at which the variability of the retrieved field matches that of a reference field (quantified in our previous work as the scale at which the spectral energy of the error is less than half of the spectral energy that of the reference field; Guilloteau et al. 2017).
This research has two main objectives: (1) to develop a methodology to constrain the inversion at the pixel level by incorporating neighboring information from the spatial patterns and covariation of TBs and by explicitly constraining the spatial consistency of the retrieved precipitation fields; and (2) to implement the developed methodology in the current version of GPROF and quantify the uncertainty of the retrievals and the scale-dependence and spatial correlation of the retrieval error for more accurate merging of multi-satellite observations. Improved retrieval accuracy and resolution are expected to better capture extremes for hydrologic and hazard applications.
To avoid the dramatic increase of the dimension of the inverse problem by including information from surrounding pixels, we plan to strategically and systematically investigate various methods to derive a limited number of informative nonlocal parameters and incorporate them in the observation vector for the retrieval. Since the observed TBs at different channels are not only correlated to each other but also spatially correlated, they offer the opportunity to reduce the spatial information into a smaller number of uncorrelated parameters. The challenge is to determine the minimal set of these discriminatory parameters and enrich the information content of the observation vector to allow a more accurate inversion while preserving the existing dictionary-based Bayesian approach of the GPROF algorithm.
NSF: Water Sustainability and Climate - REACH (REsilience under Accelerated CHange)
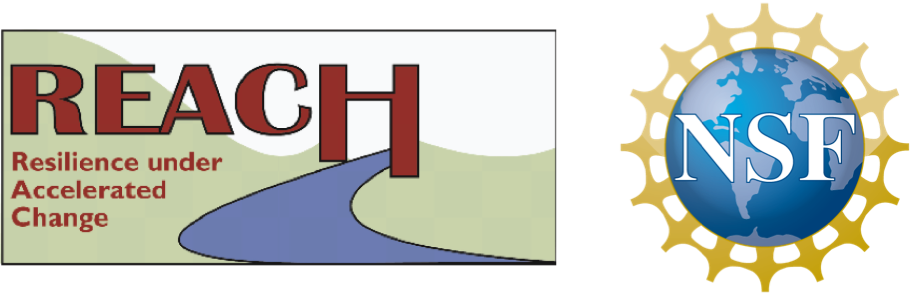
REACH (REsilience under Accelerated CHange) is a multi-institution, interdisciplinary effort led by the University of Minnesota, with the overall goal to develop a "framework" within which the vulnerabilities of a natural-human system can be assessed to guide decision-making towards sustainability and resilience. A unique element of the framework is identifying and focusing on places, times, and processes of accelerated or amplified change. One specific hypothesis to be tested is that of Human Amplified Natural Change (HANC), which states that areas of the landscape that are most susceptible to human, climatic, and other external changes are those that are undergoing the highest natural rates of change. To test the HANC hypothesis and turn it into a useful paradigm for enabling water sustainability studies, a predictive understanding of the cascade of changes and local amplifications between climatic, human, hydrologic, geomorphologic, and biologic processes are being developed to identify "hot spots" of sensitivity to change and inform mitigation activities.
The developed framework is being tested in the Minnesota River Basin (MRB) where pervasive landscape disturbances due to geological history and human actions are affecting changes in ecosystem health and water quantity and quality. Underlying our research efforts are the following science questions (hypotheses): (1) What are the major drivers of change in the MRB, and how do natural and anthropogenic changes interact, propagate and amplify across scales and across processes (physical, biological, and socio-economic)? (2) How can the history and present state of landscape organization inform the identification of "hot spots" of change? (3) What modeling frameworks (from fully distributed to reduced complexity network-based models) can capture the cascade of physical, biological, and socio-economic changes in data-limited environments and for short to long-term predictions? and (4) How can policy and management decisions be informed by understanding the system vulnerabilities and the places/times most sensitive to change? In other words, how can we preserve and improve water quality, ecosystem functioning, and resilience while still meeting the needs of ecosystems and society?
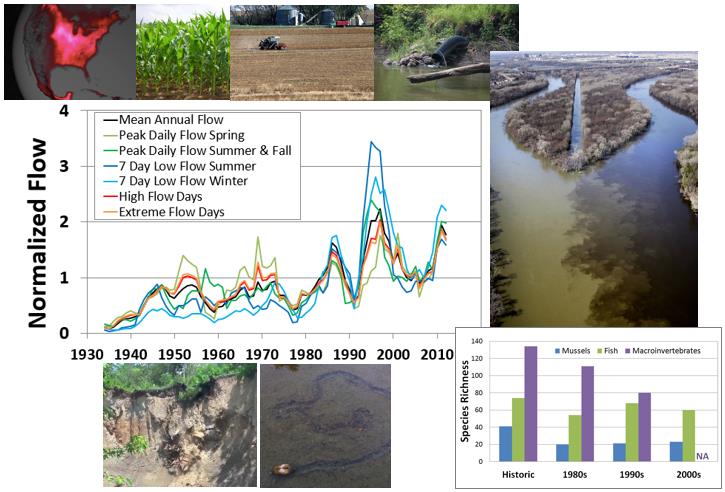
A schematic of the challenges associated with the Minnesota River Basin (MRB) highlighting the intense crop productivity during the growing season, the artificial drainage that has contributed to an accelerated hydrologic cycle, the geologic legacy of the landscape that predisposes it to increased sediment production and the eventual cascade of these changes to diminishing aquatic species richness in many streams of the basin.
Collaborators: Jacques C. Finlay (Univ. of Minnesota), Karen B. Gran (Univ. of Minnesota - Duluth), Gillian H. Roehrig (Univ. of Minnesota), Patrick Belmont (Utah State Univ.), Peter R. Wilcock (Johns Hopkins University now at Utah State Univ.), Gary Parker (Univ. of Illinois at Urbana-Champaign), Praveen Kumar (Univ. of Illinois at Urbana-Champaign), Catherine L. Kling (Iowa State Univ.), Sergey Rabotyagov (Univ. of Washington).
NSF Water Sustainability and Climate (WSC) project EAR-1209402.
Project Report Year 1
Project Report Year 2
Project Report Year 3
Project Report Year 4
Project Report Year 5
Project Report Year 6
Project Outcomes Report
NSF-Belmont Forum: DELTAS project - Catalyzing action towards sustainability of deltaic systems with an integrated modeling framework for risk assessment
Deltas are dynamic landforms at the land-ocean boundary, involving intricate mazes of river channels, estuarine waterways, and vast, often flooded landscapes. They cover 1% of Earth, yet are home to over half a billion people. Deltas sustain biodiverse and rich ecosystems, such as mangroves, reedlands and marshes. They are also economic hotspots that support major fisheries, forest production, and agriculture, as well as major urban centers, ports, and harbors. Yet, worldwide delta systems, including the people, economies, infrastructure, and ecology they support, are under threat from a range of natural and anthropogenic activities.
The DELTAS project, led by the University of Minnesota, is funded by the Belmont Forum to develop a science-based integrative modeling framework that can be used to assess delta vulnerability and guide sustainable management and policy decisions at the regional and local scales. The DELTAS project will investigate several key questions in delta functioning and vulnerability, towards proposing sustainable solutions. These questions include: (1) How do climate change, pressure on resources, and engineering/infrastructure development make the inhabitants, biodiversity, and ecosystems of deltas vulnerable? (2) How is this vulnerability to be measured? (3) How do delta areas absorb extreme events? What are the hydrological and ecological thresholds underlying the integrity of a delta region? (4) What are the relevant local and regional biophysical and social stressors for a particular delta system, how do these interact, and how do they vary spatially and over time? (5) How can regional delta sustainability be balanced with economic growth? and (6) How can one reduce future risk while attaining sustainable development?
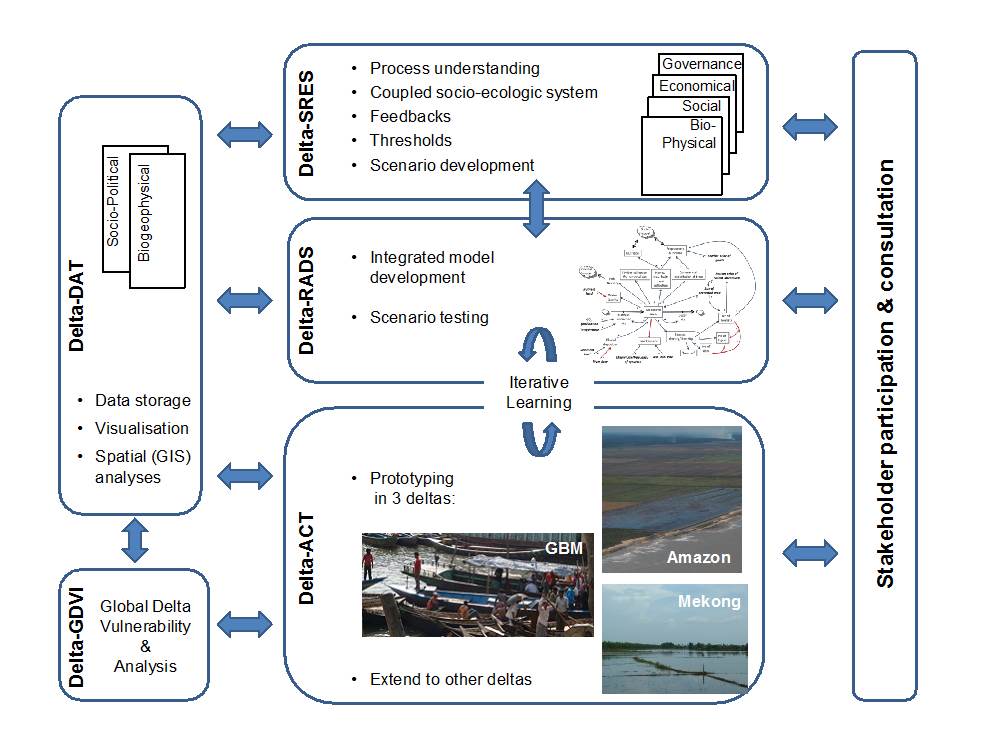
Our research framework comprises five different components:
Delta-SRES: Develop a theoretical framework for assessing delta vulnerability and the possibility for transitions to undesired biophysical or socio-economic states under various scenarios of change.
Delta-RADS: Develop an open-access, science-based, integrative modeling framework called the Delta Risk Assessment and Decision Support (RADS) Tool. This tool will be a GIS modeling system that will support quantitative mapping and definition of functional relationships of the bio-physical environment of deltas as well as their social and economic dynamics.
Delta-DAT: Consolidate data on bio-physical, social, and economic parameters into an international repository of integrated data sets and make these readily available relevant data for use by the community at large to assess critical parameters, compute vulnerability metrics, and provide input data to the Deltas-RADS modeling framework.
Delta-GDVI: Develop Global Delta Vulnerability Indices (GDVI) that capture the current and projected physical-social-economic status of deltas around the world ("delta vulnerability profiles").
Delta-ACT: Work with regional teams and stakeholders to put the products of Delta-SRES, Delta-RADS and Delta-DAT into action by demonstrating the implementation of the developed framework to three major deltas. The selected deltas are the Ganges-Brahmaputra-Meghna (GBM), Mekong, and Amazon deltas.
Amazon River Delta (ARD). The ARD delta is the world's largest delta (~450,000 km2) influencing the coastal economies of Brazil, French Guiana, Surinam, Guyana and Venezuela. In terms of environmental challenges that are typically associated with delta systems, the ARD is often classified as low risk because of its limited damming and water/oil extraction. However, deforestation proceeds at a rapid pace, and population, economy, and infrastructure in Amazonia are growing quickly. Ultimately, like all deltas, the ARD faces multiple, imminent environmental threats.
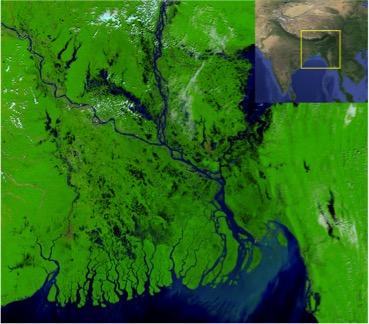
Ganges-Brahmaputra-Meghna (GBM) Delta. The GBM delta is the world's second largest delta (~100,000 km2) draining land from Bangladesh, Bhutan, China, India and Nepal. The delta covers most of Bangladesh and part of West Bengal, India, with many of the 147 million people (in 2000) living in the delta under extreme poverty and facing multiple challenges. The population is expected to increase by 28% in 2015. Already 30% of Bangladesh is within 5 m of sea level, experiencing tidal water movement 100 km inland during the dry season; and relative sea-level rise that exceeds global mean sea-level rise, demonstrating subsidence. Together these factors make the GBM delta one of the most vulnerable coastal regions in the 21st century.
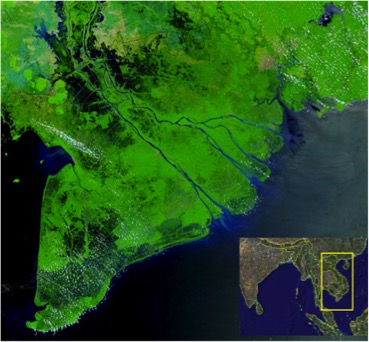
Mekong River Delta (MRD). The MRD is the world's third largest delta (~50,000 km2) and considered as one of Asia's main food baskets. The major challenges in the MRD can be attributed to socio-economic transformation and urbanization processes leading to the degradation of natural forests and wetland areas, accompanied by increasing water pollution. The MRD is now undergoing large-scale erosion, especially in the muddy mangrove-rich western part, increasing its vulnerability under projected sea-level rise and impacting future food security. Other activities include large-scale sand mining in the river and delta reaches, mangrove removal for shrimp farms, dikes and embankments to protect shrimp farms from flooding, and future large-scale hydropower development upstream. The fact that the Mekong river catchment is shared among six countries (China, Myanmar, Lao PDR, Thailand, Cambodia and Vietnam) is a potential source of conflict in harnessing the resources of the basin, especially hydropower development.
Participating countries: USA, India, Japan, Canada, Germany, France, Norway, China, UK, Netherlands, Vietnam, and Bangladesh.
Partner Institutions: University of Minnesota (lead institution, USA), University of Dhaka (Bangladesh), Aix-Marseille Univ. (France), Indiana University (USA), Deltares (Netherlands), German Aerospace Center (Germany), World Wide Fund for Nature - Greater Mekong Program (Vietnam), Vanderbilt University (USA), International Union for the Conservation of Nature (USA), United Nations Environment Programme (France), Univ. of Southampton (UK), Vietnam Academy of Science and Technology (Vietnam), University of Colorado, Boulder (USA), Anna University (India), United Nations University (France), Geological Survey of Japan (Japan), Bangladesh Univ. of Engineering and Technology (Bangladesh), Norwegian Institute for Air Research (Norway), Natural Resources Canada (Canada), University of Waterloo (Canada), and Nanjing University (China).
Belmont Forum and G8 Research Councils Initiative on Multilateral Research Funding - International Opportunities Fund G8MUREFU3-2201-037.
Project Report Year 1
Project Report Year 2
Project Report Year 3
Project Report Year 4
Project Report Year 5
Project Outcomes Report
NSF: International Collaboratory - LIFE (Linked Institutions for Future Earth)
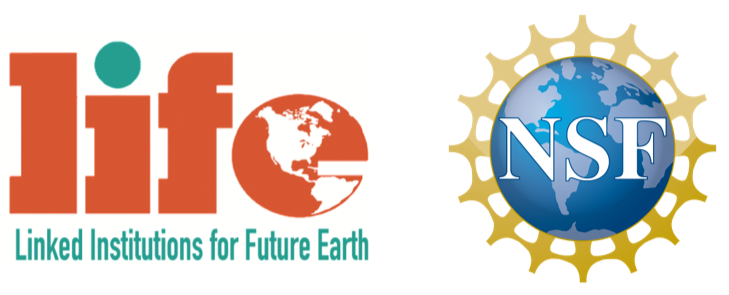
Among the many dimensions of the accelerating pace of global environmental change, the Earth's surface - the environment where most life and human activity evolves - is perhaps the most central but until recently among the least comprehensively studied. The Earth's surface is undergoing profound change due to human activities (land-use change, intensive agriculture to feed the world's increasing population, urbanization, etc.), natural hazards caused by increased extreme events in a warming climate (landslides, droughts, floods), and sea level rise causing coastal erosion and ecosystem degradation in deltas around the world. Building on the success of the National Center for Earth surface Dynamics (NCED) in providing a national collaboratory for Earth surface dynamics research, this project extends our efforts internationally by establishing a Virtual Institute called LIFE (Linked Institutions for Future Earth). This virtual institute aims at coordinating international research built on sharing unique experimental facilities, theoretical strengths, and field observations for advancing the quantitative, predictive understanding of the Earth surface system and its response to change.
Collaborators: Chris Paola (Univ. of Minnesota), Vaughan Voller (Univ. of Minnesota), William Dietrich (Univ. of California - Berkeley), Paola Passalacqua (Univ. of Texas - Austin), Praveen Kumar (Univ. of Illinois), Patrick Hamilton (Science Museum of Minnesota), Vladimir Nikora (Univ. of Aberdeen), Liam Reinhardt (Univ. of Exeter), Francois Metivier (Institut de Physique du Globe de Paris), Antonio Parodi (CIMA Research Foundation), Daniel Conde (Universidad de la Republica - Uruguay), Cristian Escauriaza (Pontifica Universidad Catolica de Chile), Rina Schumer (Desert Research Institute), Laurel Larsen (Univ. of California - Berkeley).
NSF Science Across Virtual Institutes (SAVI) project EAR-1242458.
Project Report Year 1
Project Report Year 2
Project Report Year 3
Project Report Year 4
Project Report Year 5
Project Report Year 6
NASA: Next Generation of Multi-sensor Multi-scale Precipitation Fusion Products for the GPM era
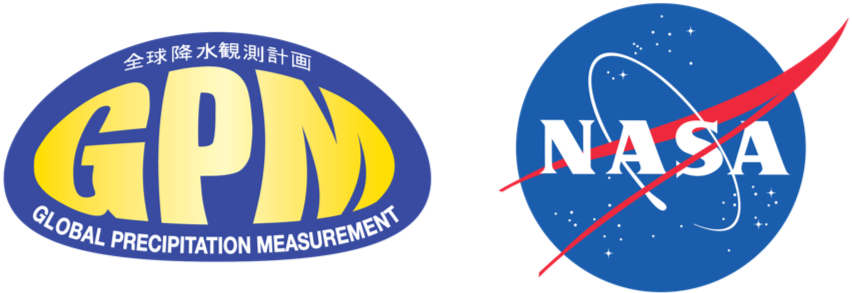
We develop a new and innovative formalism for statistical estimation (fusion, retrieval, and resolution enhancement) of multi-sensor, multi-scale precipitation measurements in the GPM era and steps for operational implementation. We pose the fusion problem in a nonlinear variational formulation setting and use a Maximum a Posteriori (MAP) estimation which is constrained by a sparse prior in the wavelet domain. In contrast to the widely used least-squares approaches which yield smoother estimates, the proposed approach incorporates a non-smooth term (L1 norm) and, as such, allows preservation of extremes and localized features. Within the same general formalism, we propose new ideas for improving the performance of precipitation retrieval and resolution enhancement of multi-sensor products using a non-linear dictionary-based inverse estimation approach with sparse priors. The ultimate goal of the proposed research, in collaboration with NASA researchers, is to develop state-of-the-art and operationally viable algorithms for optimal fusion, downscaling, and retrieval of multi-instrument and multi-satellite precipitation data for enhanced utilization of the TRMM/GPM products in climate, weather, and hydrologic prediction studies.
NASA Precipitation Measurement Missions Science Team
|